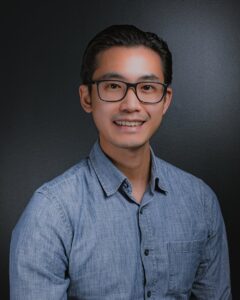
Researchers in Canada have developed machine learning (ML) models that they claim, “can accurately predict one-year IVC [inferior vena cava] filter complications, performing better than logistic regression”.
Writing in the Journal of Vascular Surgery: Venous and Lymphatic Disorders (JVS-VL), Ben Li (University of Toronto, Toronto, Canada) and colleagues highlight that IVC filter placement is associated with long-term complications and posit that predictive models for filter-related issues “may help guide clinical decision-making”. The authors state that it was their objective to develop ML algorithms that predict one-year IVC filter complications using preoperative data.
Li et al write that they used the Vascular Quality Initiative (VQI) database to identify patients who underwent IVC filter placement between 2013 and 2024. They identified 77 preoperative demographic and clinical features from the index hospitalisation when the filter was placed.
The authors note that the primary outcome of the study was one-year filter-related complications, specifically a composite of filter thrombosis, migration, angulation, fracture, and embolization or fragmentation, vein perforation, new caval or iliac vein thrombosis, new pulmonary embolism, access site thrombosis, or failed retrieval.
Going into more detail regarding their study methods, Li and colleagues share that the data were divided into training (70%) and test (30%) sets, and that six ML models were trained using preoperative features with 10-fold cross-validation. These were Extreme Gradient Boosting, random forest, Naïve Bayes classifier, support vector machine, artificial neural network, and logistic regression. The primary model evaluation metric was area under the receiver operating characteristic curve (AUROC).
The researchers also assessed model robustness, detailing that they used calibration plot and Brier score to do so, and evaluated performance across subgroups based on age, sex, race, ethnicity, rurality, median Area Deprivation Index, planned duration of filter, landing site of filter, and presence of prior IVC filter placement.
Li et al report in JVS-VL that 14,476 patients underwent IVC filter placement over the course of the 11-year study period, of whom 584 (4%) experienced one-year filter-related complications.
“Patients with a primary outcome were younger and more likely to have thrombotic risk factors including thrombophilia, prior venous thromboembolism (VTE), and family history of VTE,” the authors detail.
Furthermore, Li and colleagues reveal that the best prediction model was Extreme Gradient Boosting, achieving an AUROC of 0.93. Comparatively, they continue, logistic regression had an AUROC of 0.63.
The authors state that calibration plot showed “good agreement” between predicted and observed event probabilities with a Brier score of 0.07.
In addition, Li et al report that that top 10 predictors of one-year filter-related complications were, in order, thrombophilia, prior VTE, antiphospholipid antibodies, factor V Leiden mutation, family history of VTE, planned duration of IVC filter (temporary), unable to maintain therapeutic anticoagulation, malignancy, recent or active bleeding, and age. “Model performance remained robust across all subgroups,” the researchers share.
In their conclusion, Li and colleagues posit that the ML algorithms assessed in the present study “have potential to guide patient selection for filter placement, counselling, perioperative management, and follow-up to mitigate filter-related complications and improve outcomes”.